The Role of Machine learning in Evolving Semiconductor Package Design and Verification
The semiconductor industry is changing fast, with growing needs for speed, smaller size, and energy efficiency. As packages become more complex, old design methods can’t keep up. That’s where machine learning (ML) comes in, bringing a game-changing solution. ML uses data to help companies make and design chips better and faster, solving today’s challenges.
In this blog, we’ll explore how machine learning is evolving semiconductor package design, with practical examples showing its impact.
The Growing Complexity of Semiconductor Package Design:
A semiconductor package connects the silicon chip to the rest of the system, typically on a printed circuit board (PCB). The package handles power distribution, signal integrity, and heat dissipation, all of which must be managed effectively to ensure optimal performance. However, as chips become more advanced, the design process faces challenges in managing complex variables like thermal behaviour, power efficiency, and electromagnetic interference (EMI).
To address these challenges, PCB design companies and chip manufacturers are increasingly turning to machine learning for optimization, fault detection, and accelerated verification.
Machine Learning: A New Frontier in Semiconductor Design
Machine learning, a subset of artificial intelligence, excels at recognizing patterns in vast amounts of data and making data-driven decisions. This makes it an ideal tool for semiconductor design, where there is a need to analyze historical data, optimize workflows, and predict outcomes in complex environments. ML is already helping semiconductor manufacturing companies by automating layout design, enhancing fault detection, and optimizing thermal and power management. By leveraging historical data from previous designs, simulations, and real-world performance metrics, machine learning models can predict and improve the performance of future designs, significantly reducing manual labour and time spent in iterative processes.
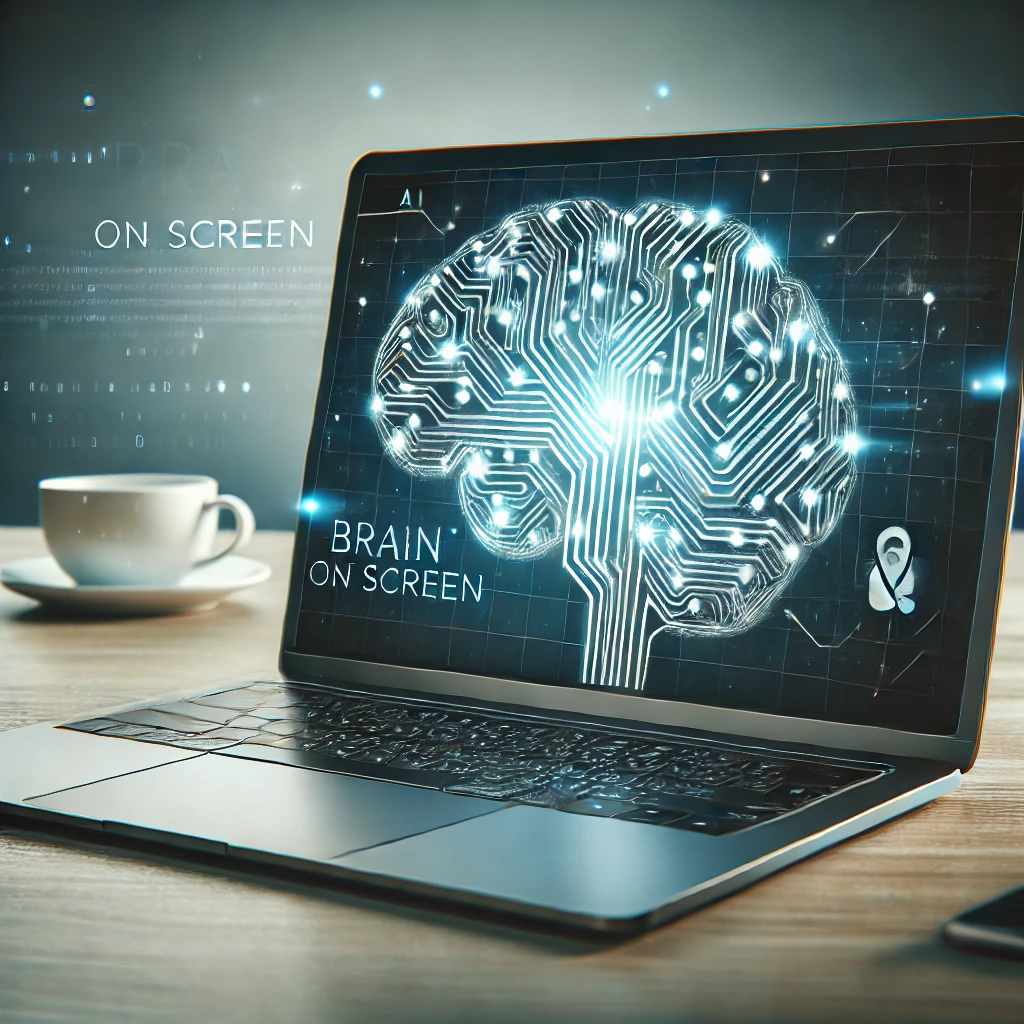
Practical Applications of Machine Learning in Semiconductor Package Design
1. Design Optimization with Historical Data
Machine learning optimizes semiconductor package design by streamlining component placement and routing paths. Intel and Nvidia utilize ML models to analyze historical design data, recommending ideal layouts. This approach reduces iterations and accelerates development.
A case study showcases ML’s impact on a complex GPU design:
– Trained ML model on prior design data
– Reduced routing congestion by 25%
– Improved signal integrity by 30%
– Faster design turnaround
– Enhanced performance
2. Fault Detection and Yield Improvement
Finding faults during chip production is vital to ensure most chips work properly. Traditional methods rely on manual checks or fixed rules, but machine learning (ML) does it better:
1. Analyses sensor data in real-time.
2. Detects defects automatically.
A Taiwanese chip maker used ML to monitor production sensors. The model spotted tiny temperature and pressure changes, predicting potential faults before they caused harm.
Result:
– 5% more functional chips produced.
– Less production downtime.
3. Thermal and Power Management
Machine learning helps chip makers manage heat and power in semiconductor packages. As chips get smaller and more complex, heat buildup is a major issue. ML solves this by:
1. Simulating thermal performance under different conditions.
2. Designing efficient cooling solutions.
A smartphone manufacturer used ML to optimize power consumption in their new processors. The ML model analyzed power data, enabling real-time adjustments that:
1. Improved battery life.
2. Maintained performance.
Result: Smarter, more efficient chip design.
4. Accelerating Verification and Testing
Verifying chip designs is a lengthy process that ensures everything works as planned. Machine learning speeds this up by:
1. Identifying high-risk areas to focus on.
2. Skipping unnecessary tests using past data. A practical case involves a chip manufacturer used AI to predict and skip redundant tests, reducing verification time by 30% while maintaining quality. This saved time and let engineers work on more important tasks.

5. Predictive Maintenance for Manufacturing Equipment
In chip manufacturing, precise control is crucial for quality and efficiency. Tiny changes in temperature, pressure, or materials can cause defects. Machine learning helps by:
1. Monitoring sensor data in real-time to predict potential issues.
2. Alerting manufacturers to take corrective action before problems arise.
Additionally, ML enables predictive maintenance by analysing equipment data to:
1. Forecast machine failures.
2. Schedule proactive maintenance.
This minimizes downtime and keeps production on track.
Real-World Benefits
The integration of machine learning brings numerous advantages:
– Faster Time-to-Market: Automated design optimization accelerates verification cycles.
– Enhanced Yield: Predictive maintenance and fault detection minimize defects.
– Optimized Thermal Management: Improved designs enable efficient cooling solutions.
Industry leaders like Intel, AMD, and TSMC are leveraging ML-driven design processes, paving the way for:
– Next-generation applications
– Increased innovation
– Improved performance
Unlock the potential of machine learning in semiconductor package design.
Conclusion
As semiconductor packaging becomes more complex, machine learning is playing an increasingly critical role in helping PCB design companies and semiconductor manufacturing companies manage this complexity. From optimizing design layouts to enhancing fault detection and accelerating verification, ML offers a wide range of benefits. With the integration of machine learning, chip manufacturers are not just keeping pace with the rapid evolution of technology—they’re driving it forward. In the years to come, we can expect machine learning to become even more embedded in the semiconductor design and verification process, unlocking new possibilities for innovation.